Improving your marathon with the wisdom of the dataset
UCD and Insight researchers Professor Padraig Cunningham and Professor Barry Smyth have developed a data science approach to achieving a personal best in a marathon
“How do you know someone has run a marathon?” asks Professor Barry Smyth, a computer scientist and keen runner himself. “Just wait, they will tell you soon enough.”
It’s true, marathon runners can often display an enthusiastic zeal about their sport and they are justifiably proud of their hard-earned race times. In the run up to the Dublin Marathon this October, athletes pore over training plans, tracking their mileage and tailoring their fitness to that all important deadline and finish line.
But what if they could get a helping hand from data science? Professor Smyth and Professor Cunningham, UCD School of Computer Science, recently developed a method to plumb datasets of archived marathon race times and use software to work out ways to realistically improve an individual runner’s personal best time.
In June the two professors scooped the Best Paper award at the 25th International Conference on Case-Based Reasoning in Trondheim, Norway, and what particularly impressed the judges was the novelty of applying data analysis methods to marathon data.
The case of the better marathon time
To carry out the study the UCD researchers, who are both Founding Directors at the Science Foundation Ireland -funded Insight Centre for Data Analytics, analysed publicly available race times from previous London Marathon races. Their approach, a type of machine learning called case-based reasoning, allowed them to learn from the experience of individual runners who improved their times over subsequent races.
“The idea behind case-based reasoning is to re-use experiences in order to have better outcomes for new challenges,” explains Professor Cunningham, who is Professor of Knowledge and Data Engineering at UCD. “It is a problem-solving idea, so if I have a problem that is similar to a problem that somebody else has encountered in the past, I get to re-use their solution.”
The ‘problem’ in this case is the desire to improve your race time and achieve a new personal best. And the previous problems (so-so race times) and solutions (better race times) lie within more than 200,000 races recorded over six years of the London Marathon.
Within those datasets lie patterns where an individual has run a so-so race and then gone on to achieve faster times in one or more subsequent races. By analysing the baseline and improvements, the new algorithm can help you optimise your own race time over that course.
Optimal guidance
The idea for the study came when Professors Cunningham, a keen cyclist, and Professor Smyth, a marathon runner, were out for a walk. They had both worked in the area of case-based reasoning for more than two decades and they got to discussing how they could apply the technique to optimise athletic performance.
“About 18 months ago I started analysing marathon data,” says Professor Smyth, who holds the Digital Chair of Computer Science at UCD. “I started downloading the results datasets of marathons, digging around in the data and writing blog posts and magazine articles for the running community. Then myself and Pádraig (Cunningham) started talking about whether you could work out how to improve your personal best time on the basis of other runners who have already done that.”
But setting that personal best goal isn’t just a case of plucking a number out of the air, notes Professor Smyth. “If I pick something too hard I am likely to blow up during the race, so you want that sweet spot where you can achieve it,” he says. “You also want some guidance on the optimal way to run the race, the target split times over 5k segments.”
Baseline to better
The UCD researchers developed a method to mine the London data for baseline and improved races, allowing an individual runner to ‘twin’ their baseline race with a group of individuals who had run similar initial races and then get pointers on how to improve towards a realistic personal best.
“It is different from the programmes that predict your marathon time based on your 10k or half-marathon times,” explains Professor Smyth. “This is much more personalised and it takes the topology of the race into account, giving you optimal splits along the way.”
The approach is a ‘big data’ version of going and talking to runners who have had similar races to your first one and asking them about your experience, notes Professor Cunningham. Rather than you seeking out your ‘twin’ runner who has gone on to better times, the software does the heavy lifting through the dataset to find those patterns, then it delivers the optimised times.
This ability to optimise based on past patterns plugs into a larger trend in data science, he adds. “At the moment, we can use data to predict future events but the next generation of systems will go beyond just predicting outcomes and focus on optimising outcomes – what is the best outcome we can have and how can we get there?”
Optimising the future
Looking to how the work from this case-based reasoning paper could further develop, Professor Smyth describes how marathon apps could deploy it on their sites. “Rather than having a one-size-fits-all prediction tool, you could enter your running times and get an optimal race, a set of targets.”
A further iteration of the technology could track you as you run the race and alter the optimal split times depending on how the race is going, he adds. “A guide to how to run the race on the day is one thing, but what happens in the middle of the race when it hasn’t gone so well – you might have another bit of AI (artificial intelligence) which is adjusting your goals.
The approach could dig even deeper if applied to training data in running and cycling. “A piece of artificial intelligence could tweak your training programme, even picking up on early signs of overtraining and helping you to avoid injury,” says Professor Smyth.
Professors Pádraig Cunningham and Barry Smyth were in conversation with Dr Claire O’Connell, science writer and contributor to The Irish Times and Silicon Republic
This article first appeared in the Autumn 2017 edition of UCD Today
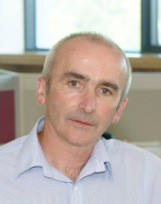
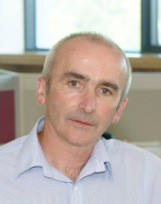
Featured Researcher:
Professor Padraig Cunningham
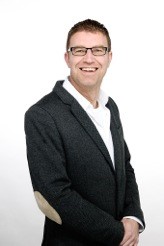